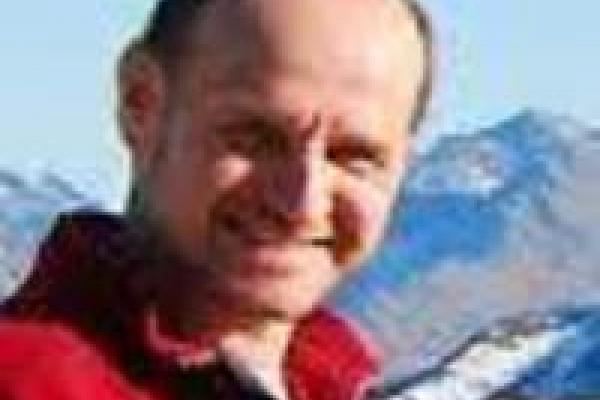
Seminar Series: Quantum Information
Friday, January 10
Physics Research Building, Room 4138
Maciej Maska, University of Silesia, Poland
Title: Learning by Confusion Identification and Characterization of Phase Transitions
Abstract: The conventional methods of classification of phase transitions rely on identification of order parameters and singularities in the free energy and its derivatives. Recently, artificial neural networks have been proven to be an efficient tool to perform this task. It has been shown that properly trained neural network can precisely determine the critical temperature. The most popular approach is based on supervised learning, where the network is trained on a large number of labeled configurations. Another approach, "learning by confusion" is a combination of supervised and unsupervised learning. I will show how these methods can be used to identify phase transitions in simple condensed matter models. Additionally, I will demonstrate how the confusion scheme can be exploited to determine the order of a phase transition.
Event is free and open to the public.